Artificial intelligence has modified the way in which science is finished by allowing researchers to research the large amounts of knowledge modern scientific instruments generate. It can discover a needle in 1,000,000 haystacks of knowledge and, using deep learning, it could actually learn from the information itself. AI is accelerating advances in gene hunting, medicine, drug design and the creation of organic compounds.
Deep learning uses algorithms, often neural networks which can be trained on large amounts of knowledge, to extract information from latest data. It could be very different from traditional computing with its step-by-step instructions. Rather, it learns from data. Deep learning is much less transparent than traditional computer programming, leaving necessary questions – what has the system learned, what does it know?
As a chemistry professor I wish to design tests which have at the very least one difficult query that stretches the scholars’ knowledge to ascertain whether or not they can mix different ideas and synthesize latest ideas and ideas. We have devised such an issue for the poster child of AI advocates, AlphaFold, which has solved the protein-folding problem.
Protein folding
Proteins are present in all living organisms. They provide the cells with structure, catalyze reactions, transport small molecules, digest food and do rather more. They are made up of long chains of amino acids like beads on a string. But for a protein to do its job within the cell, it must twist and bend into a posh three-dimensional structure, a process called protein folding. Misfolded proteins can result in disease.
In his chemistry Nobel acceptance speech in 1972, Christiaan Anfinsen postulated that it must be possible to calculate the three-dimensional structure of a protein from the sequence of its constructing blocks, the amino acids.
Just because the order and spacing of the letters in this text give it sense and message, so the order of the amino acids determines the protein’s identity and shape, which ends up in its function.
Marc Zimmer, CC BY-ND
Because of the inherent flexibility of the amino acid constructing blocks, a typical protein can adopt an estimated 10 to the ability of 300 different forms. This is an enormous number, greater than the variety of atoms within the universe. Yet inside a millisecond every protein in an organism will fold into its very own specific shape – the lowest-energy arrangement of all of the chemical bonds that make up the protein. Change only one amino acid within the tons of of amino acids typically present in a protein and it could misfold and not work.
AlphaFold
For 50 years computer scientists have tried to unravel the protein-folding problem – with little success. Then in 2016 DeepMind, an AI subsidiary of Google parent Alphabet, initiated its AlphaFold program. It used the protein databank as its training set, which comprises the experimentally determined structures of over 150,000 proteins.
In lower than five years AlphaFold had the protein-folding problem beat – at the very least essentially the most useful a part of it, namely, determining the protein structure from its amino acid sequence. AlphaFold doesn’t explain how the proteins fold so quickly and accurately. It was a significant win for AI, since it not only accrued huge scientific prestige, it also was a significant scientific advance that would affect everyone’s lives.
Today, because of programs like AlphaFold2 and RoseTTAFold, researchers like me can determine the three-dimensional structure of proteins from the sequence of amino acids that make up the protein – for free of charge – in an hour or two. Before AlphaFold2 we needed to crystallize the proteins and solve the structures using X-ray crystallography, a process that took months and value tens of 1000’s of dollars per structure.
We now even have access to the AlphaFold Protein Structure Database, where Deepmind has deposited the 3D structures of nearly all of the proteins present in humans, mice and greater than 20 other species. To date they it has solved greater than 1,000,000 structures and plan so as to add one other 100 million structures this yr alone. Knowledge of proteins has skyrocketed. The structure of half of all known proteins is more likely to be documented by the tip of 2022, amongst them many latest unique structures related to latest useful functions.
Thinking like a chemist
AlphaFold2 was not designed to predict how proteins would interact with each other, yet it has been in a position to model how individual proteins mix to form large complex units composed of multiple proteins. We had a difficult query for AlphaFold – had its structural training set taught it some chemistry? Could it tell whether amino acids would react with each other – a rare yet necessary occurrence?
I’m a computational chemist eager about fluorescent proteins. These are proteins present in tons of of marine organisms like jellyfish and coral. Their glow might be used to light up and study diseases.
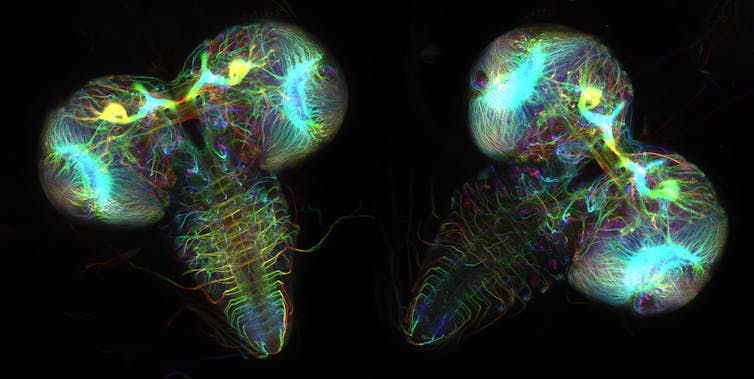
Wen Lu and Vladimir I. Gelfand, Feinberg School of Medicine, Northwestern University
There are 578 fluorescent proteins within the protein databank, of which 10 are “broken” and don’t fluoresce. Proteins rarely attack themselves, a process called autocatalytic posttranslation modification, and it is vitally difficult to predict which proteins will react with themselves and which of them won’t.
Only a chemist with a major amount of fluorescent protein knowledge would have the opportunity to make use of the amino acid sequence to search out the fluorescent proteins which have the proper amino acid sequence to undergo the chemical transformations required to make them fluorescent. When we presented AlphaFold2 with the sequences of 44 fluorescent proteins that should not within the protein databank, it folded the fixed fluorescent proteins in a different way from the broken ones.
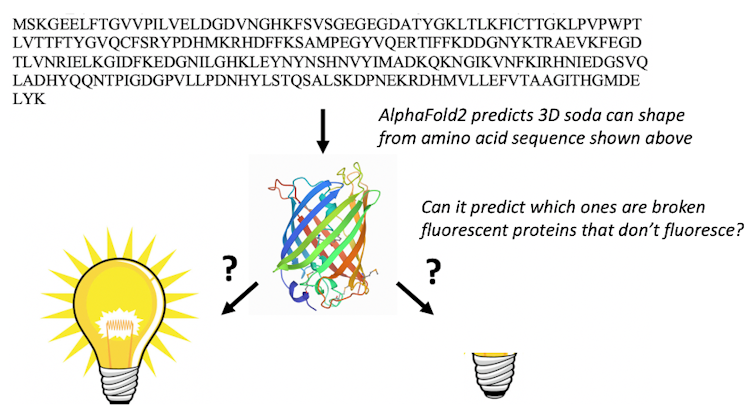
Marc Zimmer, CC BY-ND
The result stunned us: AlphaFold2 had learned some chemistry. It had discovered which amino acids in fluorescent proteins do the chemistry that makes them glow. We suspect that the protein databank training set and multiple sequence alignments enable AlphaFold2 to “think” like chemists and search for the amino acids required to react with each other to make the protein fluorescent.
A folding program learning some chemistry from its training set also has wider implications. By asking the proper questions, what else might be gained from other deep learning algorithms? Could facial recognition algorithms find hidden markers for diseases? Could algorithms designed to predict spending patterns amongst consumers also discover a propensity for minor theft or deception? And most significant, is that this capability – and similar leaps in ability in other AI systems – desirable?
This article was originally published at theconversation.com