If Health is a basic human rightHealth care is a must improved worldwide to attain universal access. However, the limited variety of doctors poses a barrier to all healthcare systems.
Healthcare approaches based on artificial intelligence (AI) are poised to fill this gap. Whether in city hospitals or in… rural and distant houses, AI has a reach that healthcare professionals cannot hope for. People searching for health information can get it quickly and conveniently. For healthcare to be effective, Patient safety must proceed to be a priority.
The news is stuffed with examples of novel applications of AI. Riding on the wave of recent interest in conversational agents, Google researchers have developed an experiment diagnostic AI, Articulate Medical Intelligence Explorer (AMIE). People in search of health information share their symptoms through a text chat interface and AMIE begins asking questions and making recommendations like a human clinician would. The researchers claim that in comparison with clinicians AMIE outperformed physicians in each diagnostic accuracy and performance.
(Google)
The potential of enormous language models (LLMs) like AMIE is obvious. By training on a big text database, LLM can generate text, discover underlying meaning, and respond in a human-like manner. Provided that patients have access to the Internet, health advice might be tailored to the patient, delivered quickly and simply, and enable triage of cases best treated by medical professionals.
However, these tools are still within the experimental stage and have limitations. AMIE researchers say more studies are needed to “imagine a future through which conversational, empathetic and diagnostic AI systems could change into protected, helpful and accessible.”
Precautions have to be taken. Providing healthcare is an advanced task. Without regulation – skilled or international – it presents challenges to quality of care, privacy and security.
Medical decision making
Medical decision-making is some of the complex and consequential activities of all. However, it could seem unlikely that an AI could work as effectively as a human clinician. Decades of research suggest that Decision-making algorithms might be equal to and even superior to clinical intuition.
Pattern recognition represents the core of medical expertise. Like other types of expertisedemand health workers extensive training to learn the diagnostic patterns, make treatment recommendations and supply care. Through effective teaching, Learners They limit the main focus of their attention to diagnostic features and ignore non-diagnostic features.
However, effective healthcare requires the next: greater than just the power to acknowledge patterns. Healthcare professionals must have the option to speak this information to their patients. Apart from the difficulties of imparting technical knowledge to patients with different levels of information Health literacyHealth information is usually emotionally charged and results in this Communication traps where doctors and patients withhold information. Through development a robust relationship with their patientsmedical professionals can fill these gaps.
The conversational features of LLMs, equivalent to ChatGPThave generated great public interest. While it’s claimed that ChatGPT “broke the Turing test” are exaggerated, their human-like reactions making LLM more appealing than before Chatbots. Future LLMs like AMIE could prove to be gaps in healthcare delivery, but they need to be used with caution.
Promise of accurate, explainable AI in healthcare
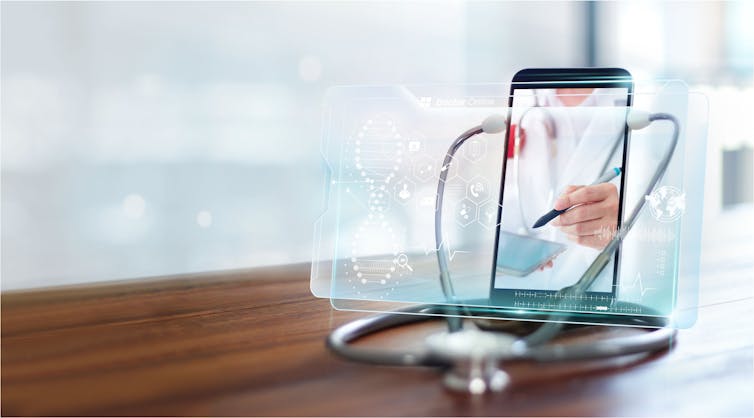
(Shutterstock)
AMIE is just not Google’s first health technology. In 2008, Google Flu Trends (GFT) was used to estimate the prevalence of influenza inside a population using aggregated search terms. They hypothesized that user search behavior must be related to flu prevalence, with past search trends predicting future cases.
GFT’s initial predictions were: quite promising. Until they failedwith old data identified as a source of bias. Later efforts to retrain the model with updated search trends again proved successful.
IBM’s Watson provides one other cautionary tale. IBM invested significant capital in the event of Watson and implemented over 50 healthcare projects. Watson’s potential didn’t come about, with the underlying technologies being quietly sold. Not only did the system fail to generate trust, that mistrust was justified when it created it “unsafe and incorrect” treatment recommendations.
AIs designed to diagnose, triage, and predict the course of COVID-19 are the perfect example of the readiness of healthcare AIs to handle public health challenges. Extensive reviews of those efforts solid doubt on the outcomes. The validity and accuracy of the models and their predictions were generally lacking. This was largely attributed to this the standard of the info.
One of the teachings to be learned from using AI during COVID is that there is no such thing as a shortage of researchers and algorithms, but there’s an urgent need for quality control. This has led to demands Design that puts people at the middle.
This also applies to expert assessments of the technologies themselves. How Google’s FRIENDMany publications evaluating these technologies are published as preprints before or in the course of the peer review process. It can be extensive Delays between a preprint and its final publication. Research has shown this and never the standard The variety of mentions on social media is a greater indicator of the download rate of a publication.
Without ensuring the validity of the training and implementation methodsHealth technologies might be introduced without formal technique of quality control.
Technology as folk medicine
The problem of AI in healthcare becomes clear once we recognize that many healthcare ecosystems can exist in parallel. Medical pluralism is observed when two or more health consumers can be found. This typically happens in the shape traditional medicine and a Western biomedical approach.
Because apps are direct-to-consumer health technologies, they represent a New folk medicine. Users adopt these technologies based on Trust quite than understanding how they work. In the absence of medical knowledge and technical understanding of how an AI works, Users must search for evidence of a technology’s effectiveness. App store reviews and suggestions can replace the expert assessment of medical professionals.
In cases where there are health concerns, users may prefer AI-powered technologies over humans stigma or chronic emotional stress. However, the accuracy of those systems could also be affected because of data update errors.
Providing user data also brings challenges. Similar to 23andMeIf users disclose personal information, clues might be left for others on their social networks.
If left unregulated, these technologies pose a challenge to the standard of care. To make sure that these technologies truly profit the general public, skilled and national regulations are needed.
This article was originally published at theconversation.com